Projects
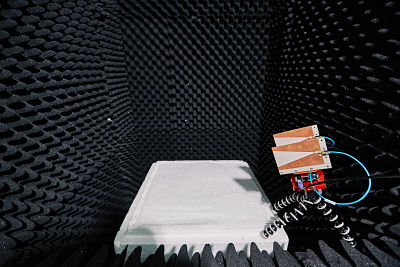
Relating snow radar retrievable parameters to snow microstructure for hydrological forecasting applications
PI: Kevin Hammonds
Ph.D. Student: Chris Donahue, Evan Schehrer
Funding Source: NASA New Investigator Program in Earth Sciences
Although terrestrial snowcovers contribute to the global water supply of nearly one-sixth of the world’s population, most of the world’s snow cover is located in remote and isolated terrain. To monitor the state of both seasonal and perennial snow covers, several snow monitoring systems based on microwave remote sensing techniques have been deployed, but a remote sensing product that derives the snow water equivalent over various types of terrain and on a global scale, has yet to be developed. In this research, the microstructural properties of snow that modify the radar reflectivity, polarimetric signature, and image coherence of multi-frequency and synthetic aperture radar systems will be investigated primarily using low power and portable ultra wideband radar systems from Flat Earth Sensing Solutions. From within the SRL, a series of controlled laboratory experiments will be performed in which artificially created snowpacks are perturbed to mimic natural-like conditions while being monitored with a full suite of remote sensing instrumentation, including multi- frequency polarimetric radar and broadband radiometry systems. To compliment these observations, advanced materials characterization techniques will also be employed, where X-ray computed microtomography, scanning electron microscopy, and electron backscatter diffraction will be used to elucidate micron and sub-micron scale snow properties such as the crystallographic orientation and the degree of anisotropy of the snow grains. With this unique combination of remote sensing and in-situ observations from within a controlled laboratory environment, new insight into the micro-scale physics of snow that drive macro-scale radar- and radiometric- observables will be derived, microwave remote sensing retrieval algorithms will be improved, and several novel approaches to the remote sensing of snow will be applied and tested.
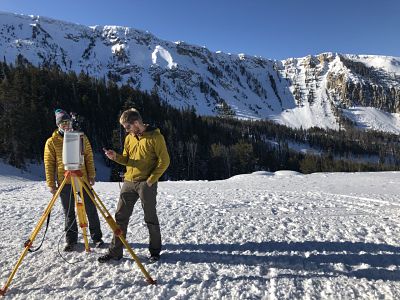
Developing Real-Time Data Processing and Analytics for Avalanche Forecasting Using Lidar
PI: Kevin Hammonds, Sean Yaw, Joe Shaw
M.S. Students: James Dillon (Civil Engineering), Peter Ottsen (Computer Science)
Funding Source: USDOT Transportation Avalanche Research Pool (TARP)
Terrestrial scanning lidar systems (TLS) have recently been shown to enhance avalanche forecasting and mitigation efforts by aiding forecasters in remotely analyzing snowpack depths over complex terrain (Deems et al. 2013; Deems et al. 2015; Prokop 2008). In this reserach, a detailed laboratory study in which both long-range and short-range TLS systems will be brought into the SRL and tested with different types of snowpacks, snow surfaces, and precipitation rates. These tests will inform what can be expected in the field and to what degree other parameters of interest can be derived. Novel analysis algorithms and software will be developed to support the use of TLS data to better inform avalanche risk assessment and decision making. Avalanche risk decision support will be driven by the extraction and aggregation of features from TLS data (e.g. snow depth, slope angles, surface properties). Algorithms will be developed that will determine these properties from streaming TLS data, such that field-users can access this data in real-time.
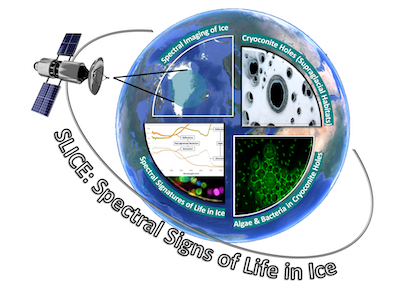
SLICE: Spectral Signs of Life in Ice
PI: Christine Foreman, MSU; Co-PI: Marco Tedesco,Lamont-Doherty Earth Observatory, Columbia University
Identifying life on other planets is one of the most exciting challenges of our times.
The Earth’s Polar Regions have long been recognized among the best terrestrial analogs
for conditions on Mars, with cryoconite holes being one of the proposed habitats for
life on other planets.Cryoconite holes are mini-entrained ecosystems, found in the ablation zone of glaciers
that provide conditions by which subsurface liquid water can exist in spite of otherwise
hostile environmental conditions.
One of the tools in the search for life has been the collection and interpretation of hyperspectral images; however the validation of reliable biomarkers in this data remains ongoing. The hyperspectral and associated measurements collected by SLICE are being used to support the analysis of data collected by theCompact Reconnaissance Imaging Spectrometer for Mars (CRISM), the OMEGA spectrometer on the Mars Express ESA mission and the THEMIS instrument on the MARS Odyssey mission. By studying the terrestrial analogs of cryoconite holes, we are isolating and culturing cryoconite organisms, determining their spectral signatures through in-situ and laboratory hyperspectral measurements and developing a spectral library of biosignatures. In this context, cryoconite holes represent a unique environment on Earth that resembles life on Mars.
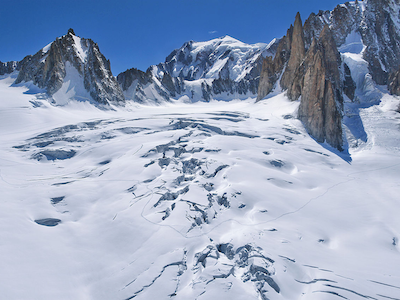
Fracture initiation and arrest in polycrstalline ice
PI: Chris Borstad
Fractures are central to a number of important climate feedbacks in glacier and ice
sheet dynamics. Crevasses at the surface of a glacier become conduits for routing
surface meltwater into the interior of the ice, where the latent-heat potential can
substantially warm and soften the ice, or all the way to the bed of the glacier, where
the water can enhance glacier sliding. Both processes can lead to enhanced glacier
motion, elevated stresses in the ice, deepening of existing crevasses, and the formation
of new crevasses, which then act to increase the catchment area for subsequent meltwater.
Such a cryo-hydrologicwarming feedback may explain some glacier surges, implying that increased glacier
surging might be expected in a warming climate. Iceberg calving from marine-terminating
glaciers is related to the presence of surface and basal crevasses in the vicinity
of the ice front. Iceberg calving laws are typically formulated as explicit boundary
conditions that specify the calving rate or the terminus position as a function of
the theoretical crevasse depth.
We have a limited understanding, from just a few experimental studies, of the fracture
properties of polycrystalline ice that govern the initiation of crevasses. However,
no studies to date have measured the conditions under which a fracture arrests, which
is relevant for correctly predicting the depth of a crevasse. The Subzero Research
Laboratory is uniquely suited to address these questions. We will create polycrystalline
ice samples in the lab and then perform standardized fracture experiments, over a
wide range of strain rates, temperatures, and grain sizes, to determine the relevant
fracture properties governing the initiation and arrest of large-scale fractures in
glacier ice. We will study the microstructure of the ice to determine where and how
fractures arrest where they do. These experiments will aid in the development of better
models for predicting crevasse depth, which will lead to more reliable predictions
of iceberg calving from glacier and ice sheet models.
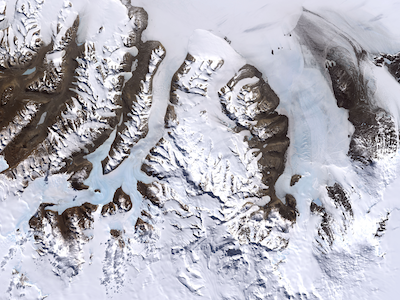
LTER: Ecosystem response to amplified landscape connectivity in the McMurdo Dry Valleys, Antarctica
PI: John Priscu
Funding: National Science Foundation
The McMurdo Dry Valleys, Antarctica, are a mosaic of terrestrial and aquatic ecosystems in a cold desert that support microbial foodwebs with few species of metazoans and no higher plants. Biota exhibit robust adaptations to the cold, dark, and arid conditions that prevail for all but a short period in the austral summer. The MCM-LTER has studied these ecosystems since 1993; during this time, we observed a prolonged cooling phase (1986-2002) that ended with an unprecedented summer of high temperature, winds, solar irradiance, glacial melt, and stream flow (the “flood year”). Since then, summers have been generally cool with relatively high solar irradiance and have included two additional high-flow seasons. Before the flood year, terrestrial and aquatic ecosystems responded synchronously to the cooling e.g., the declines in glacial melt, stream flow, lake levels, and expanding ice-cover on lakes were accompanied by declines in lake primary productivity, microbial mat coverage in streams and secondary production in soils. This overall trend of diminished melt-water flow and productivity of the previous decade was effectively reversed by the flood year, highlighting the sensitivity of this system to rapid warming. The observed lags or opposite trends in some physical and biotic properties and processes illustrated the complex aspects of biotic responses to climate variation. Since then, our conceptual model of the McMurdo Dry Valleys has evolved based on observations of discrete climate-driven events that elicit significant responses from resident biota. We now recognize that physical (climate and geological) drivers impart a dynamic connectivity among landscape units over seasonal to millennial time scales. For instance, lakes and soils have been connected through cycles of lake level rise and recession since the Last Glacial Maximum, while streams connect glaciers to lakes over seasonal time scales. Overlaid upon this physical connectivity among soils, glaciers, streams and lake are biotic linkages facilitated by the movement of genes, individuals and species through metapopulations and metacommunities. In the 5th iteration of the MCM-LTER program (MCM5) we are superimposing biotic connectivity upon this linked, heterogeneous landscape. Our hypothesis is: Increased ecological connectivity within the MDVs ecosystem will amplify exchange of biota, energy and matter, homogenizing ecosystem structure and functioning. This hypothesis will be tested with new and continuing monitoring and experiments that examine: 1) how climate variation alters connectivity among landscape units, and 2) how biota (species, populations and communities) are connected across this heterogeneous landscape using state-of-the-science tools and methods including ongoing and expanded automated sensor networks, analysis of seasonal satellite imagery, biogeochemical analyses, and next-generation sequencing. This is a six year project with current funding ending in 2023.