Assessing the Effects of Federal Money Laundering Reporting Requirements
Principal Investigator:
Joseph Atwood, Ph.D, M.S., B.S., A.A., Professor of Economics
Abstract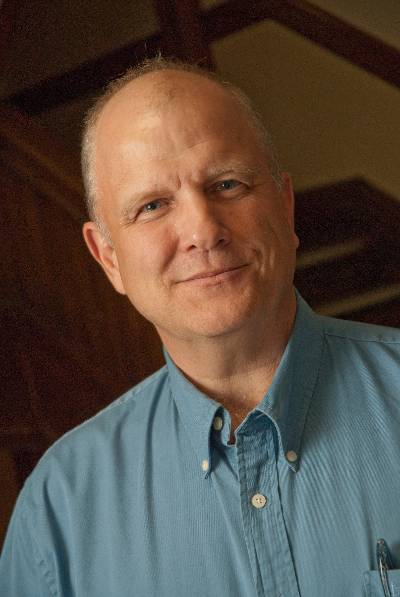
This study will examine how small, intermediate, and large banks relative efficiencies as defined above have changed through time as a result of money laundering requirements.Data from the FDIC Uniform Bank Performance Report (UBPR) data setispublicly available and contains detailed information of up to 1494 variables on over 5900 banks for the time period 2002-2017. Erika and I have processed the available data sets for the time period 2005-2017 and are in the process of identifying the potential "inputs" and "outputs" to be utilized as part of this study.
Conventional comparative DEA and qDEA "efficiency metrics" will be developed for each bank and for each year in the data set. At this time our plan is to conduct difference in differences regressions utilizing DMU's "efficiency metric" relative to level in a base period against other explanatory variables including bank size. Care will be taken in the construction/selection/normalization of the "input-output" variables to avoid/addressendogeneityand other complications in the data and estimated efficiency metrics.
Significance of the Project
This project will be focused on thetheory and the continued refinement and application of the methodology usedin assessing the comparative or relative performance or "efficiencies" of small, intermediate, andlarge-scale banks and assessing how these comparative "efficiencies" may have been effected by "money-laundering" reporting requirements.
Constructing comparative performance or 'efficiency' metrics for banks is complicated by the fact that banks perform numerous socially valuable services including consumer banking services and financial intermediation. Difficulties arise in performance assessment in that price information is not available for many of these services such as pricing the social value of check clearing services for consumers. Data envelopment analysis (DEA) is increasingly being used in assessing bank performance in that DEA methods can be implemented if the analyst can identify a set of broadly defined (and measurable) "inputs" and "outputs". DEA utilizes observed "input-output" combinations from a set of "decision making units" or DMU's to assess the ability of the set of DMU's to transform "inputs" into "outputs" and the implicit trade-offs involved in the "production process".The definition of "inputs" and "outputs" is flexible and can include non-priced characteristics such as the volume of checks processed, minority services provided, etc.
DEA utilizes observed data to construct comparative performance metrics utilizing Shepherd-related "distance functions" which measure the distance from a given DMU's observed input-output data to an "efficient-frontier" related to a "pareto-efficient-like" frontier where (holding inputs constant) output i can only be increased if output j is decreased or (holding output constant) input i can only be decreased if input j in increased, etc. With DEA, the set of observed data is used to estimate both the "frontier" and a given DMU's distance from the "efficient" frontier. This distance is broadly termed the DMU's"efficiency".We will be using a variation of this methodology i.e. quantile-DEA or qDEA.With quantile DEA distances, the "efficiency" distances are computed relative to quantile peer groups rather than computing distances to frontiers defined by "best-case" outcome realizations that may not be "reachable" by a substantial subset of the population.
It is recognized that a given firm or DMU is likely to face unobserved internal constraints that may prevent their movement to the "efficient frontier" in the short or intermediate run. As a consequence, regulators or managers may use the distance metrics as indicators of long-term potential improvements that the DMU’s operation could strive for but that attainable short term improvements may be limited.In these cases, a firm's performance will often be assessed by examining changes in their DEA "distances" over time rather than assessing their relative performance based upon the absolute levels of their "efficiency" metric at a given point in time. This is one of the approaches we plan to examine in this study in that we will contrast changes in small, intermediate, and large scale banks over time. Erika's hypothesis is that money laundering rules are likely to disproportionately and negatively affect the small banks' efficiency metrics when measured against the banking population. If this effect is realized we expect the small banks' comparative distances to increase in years after voter approved legalization of medical and/or personal marijuana use.
DEA is also useful in that the analyst can identify tradeoff rates or "implicit relative prices" between (possibly non-market traded) input-output combinations as well as determining a DMU specific "optimal" set of prices that best "support" the input-output levels observed for a specific DMU. DEA can also incorporate market price information where available and can accommodate firm or regulator specific relative trade-off preferences if desired.
The author has developed a more robust DEA procedure known as Quantile-DEA or qDEA which allows the analyst to develop comparative "efficiency" or distances to "quantile-groups" within the data set. The resulting "efficiency metrics" are both more robust to "outlier" data and enable the DMU, analyst, or regulator to compare a DMU's relative to a quantile of the population such as the top 10% or top quartile etc. TheqDEA model retains the desirable features of DEA that enable the identification of implicit prices or trade-off rates along quantile frontiers.